NEWS
Meet Mevo: The data team
Our data team is the beating heart of Material Evolution.
MevoCem, our ultra-low carbon cement, is made from industrial byproduct feedstocks, which are processed by the bespoke artificial intelligence (AI) and machine learning (ML) models that our data team have created. This innovation was recently recognised by Google via their prestigious Google for Startups AI First Accelerator, which Material Evolution is currently participating in.
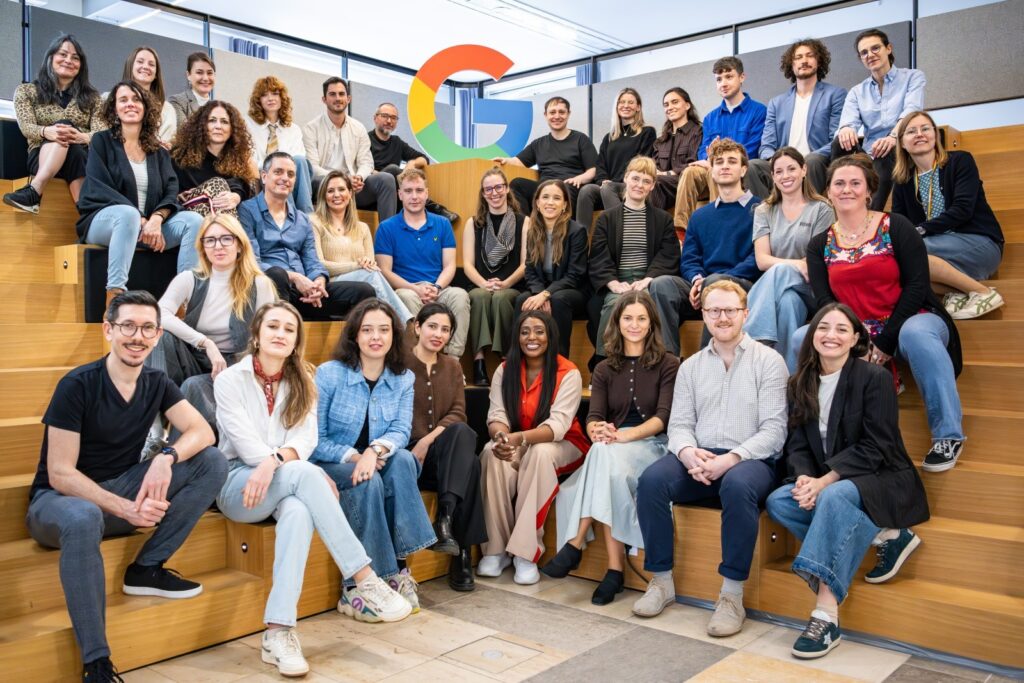
In this “Meet Mevo” installment, Sonu George, Calum Towler and James Cooper share more about their roles and career histories, the biggest challenges for implementing AI-driven solutions, and future trends for the development of AI and ML in the wider construction sector.
Read more below…
What does your career history look like and what is your role at Material Evolution?
Sonu: As a machine learning engineer at Mevo, I’m responsible for developing, training and testing a range of ML models, as well as designing and deploying applications for these models to be used by the lab team. This allows us to enhance the quality of MevoCem and accelerate the research and development process in discovering new, viable materials.
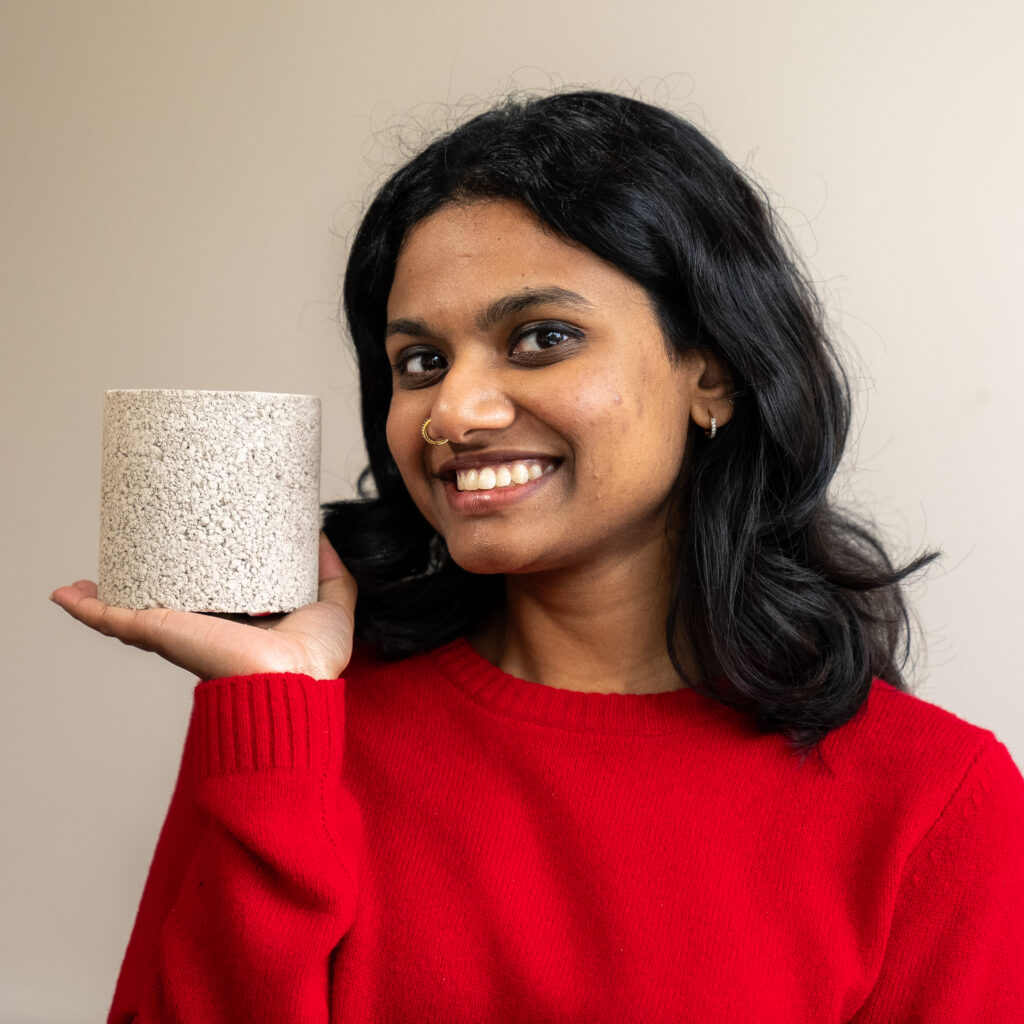
Calum: I’m a computational chemist here at Mevo, so my role is to provide a bridge between our lab and data teams. From the lab side, this involves understanding what their analytical needs are and how these can be implemented. From the data side, this involves understanding the underlying chemistry and how best to use this to improve the quality of the data science we can provide.
James: Before starting at Mevo, I worked in data and engineering roles in the financial services industry at Lloyds Banking Group and Natwest. I later joined the appointment booking platform Fresha, which taught me the potential of the data build tool (DBT) in the context of a scale-up. I then joined Mevo as a senior data engineer, and in a nutshell, my role involves building, deploying and maintaining data pipelines.
What are you working on at the moment?
Sonu: Currently, I’m working on the deployment of our Formulation Prediction Tool (FPT). This is a completely unique model that uses AI to predict how our industrial waste feedstocks will perform as ingredients for MevoCem, while recommending materials to create the ideal green cement formulation. This will help us to identify optimal waste materials in the future and reduce the need for extensive trials, making it a highly valuable tool. After designing, training and validating the model, I am now working on seamlessly integrating it across the business.
Calum: I’m in the process of starting a new project, the Treatment Prediction Tool, which will be an ML tool able to predict the effect of a given processing step, such as milling, on the fundamental material parameters.
I also help with spectroscopy services to our lab team, which is the ability to extract information from a material using electromagnetic radiation, such as visible light or X-Rays. One of my goals is to increase the accuracy and prevalence of spectroscopic analysis in our database, so that we can train predictive tools for quality control.
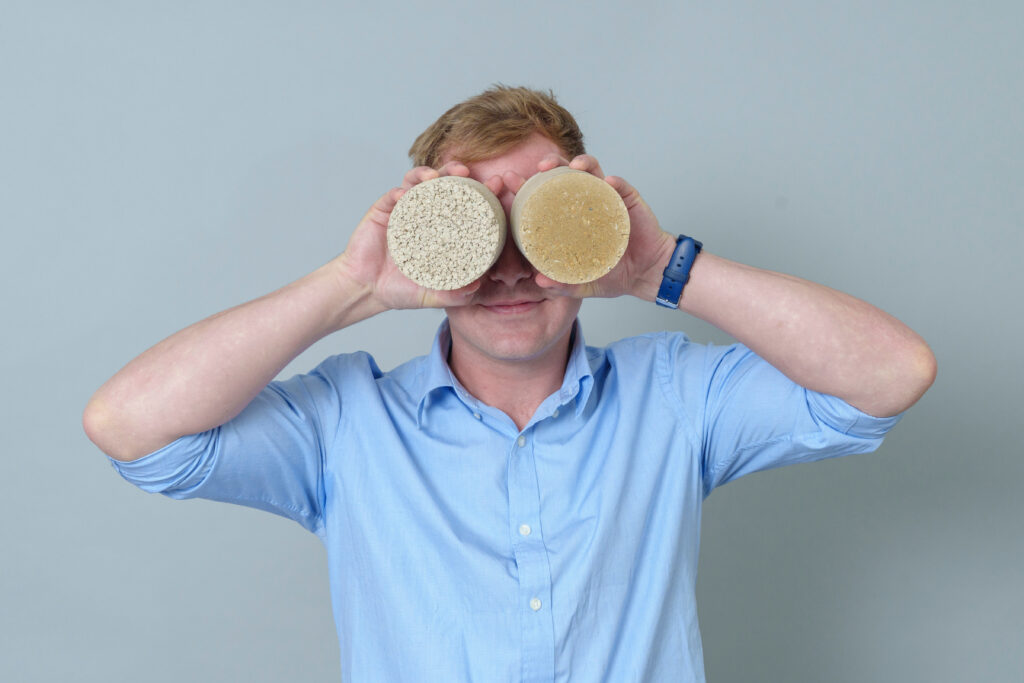
James: My role involves sourcing data from across the business, migrating it to our cloud environment and using a data build tool (DBT) to automate testing and transformation steps on the data. This ensures that what is provided back to our wider team is trusted and ready to leverage in any application that helps us – either by looking backwards at what we’ve already done (analytics) or forwards to inform what we may or may not do in future (ML).
How important is AI and ML for transforming the construction industry?
James: At Mevo, our aim is to leverage ML to enable us to streamline the development and production of novel, low carbon cements. It’s extremely important for us given that the cement industry is responsible for 8% of global carbon emissions.
Calum: AI/ML has the potential to have a big impact on optimising our novel alkali-fusion process and material science, owing to a large number of variables and properties.
Sonu: The wider adoption of AI/ML in production and supply chain management by various industries, especially during Industry 4.0, has mainly triggered the AI boom. With AI/ML showing good progress in drug discovery it was only a matter of time that it would help construction industries find new materials and tackle batch variabilities.
What are the biggest challenges when implementing AI on industrial feedstocks?
James: The variability in the input materials and data structure design.
Calum: Optimising so many variables, especially when the distribution of these variables isn’t uniform, can be challenging from both a conventional analysis and machine learning approach. We are in the process of overcoming this problem – watch this space!
The R&D cost of developing suitable formulations for new materials is very high. Reducing this will significantly mitigate one of the key challenges to replacing traditional cement manufacturing methods. It is very important, but as with all AI technology, care should be taken to ensure that the resultant analyses are used properly.
Sonu: The biggest challenge lies in real-time decision-making. Especially implementing an AI model for production lines, where the whole process is defined and runs continuously. AI being used to adjust and identify batch variabilities without the human in the loop can cause unwanted downtimes, and wrong decision-making may hurt profits. We are in the process of overcoming this problem by conducting rigorous testing of our models, feedback systems for the R&D team and continuous monitoring.
What future trends do you see in AI and ML for construction, and how can companies prepare for advancements?
James: New tools, frameworks and technologies will continue to proliferate. The companies that succeed will be those able to identify and use the subset of these tools that provide the most impact.
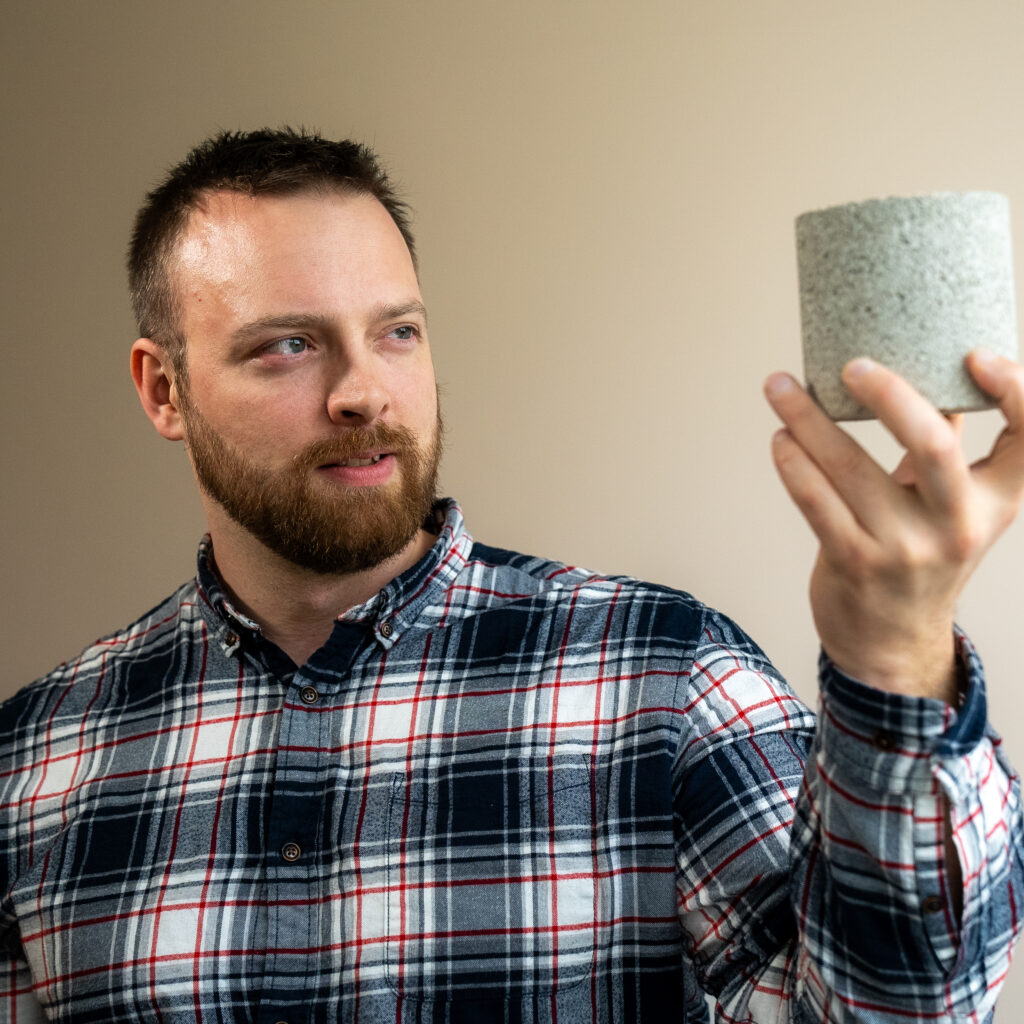
Calum: There will be increased demand for AI and ML from three sides:
- The wider market will expect these types of tools to be used
- Quality Control and Automatic Ingredient/Process Variable Adjustment
- Reducing initial product development costs
The best way any company can prepare for this demand is thorough data storage from the outset. The largest hurdle to training any model is the quality and quantity of data you can provide. Creating the required data as you go can be a lot less expensive and time-consuming than collecting it retroactively.
Sonu: Here are some areas where I think AI will be adopted soon. These are for any industry, but can be applied to the construction industry as well:
- Increased demand for end-products and less time and resources to build them can pose some challenges and fuel wider adoption.
- Balancing automation with real time needs.
- Using AI for process design and energy efficient plant operations.
- Identifying future issues, equipment monitoring and forecast possible downtime of systems.
Find our data team (Sonu, Calum and James) on LinkedIn to connect and discover more about their work.